Language Processing: The Precedence of Neural Networks on the Account of Hidden Markov Models
Authors:
Article info
2024-01-25
2024-07-19
2024-10-11
155 - 164
Keywords
- Machine learning
- Artificial Neural Networks
- Features
- Hidden Markov Models
- Natural Language Processing
- Classifiers
- Computational Linguistics
Abstract
Background: since its discovery at the beginning of the last century, Markov models gain a great popularity, and have been widely used in different domains. However, the most prominent use was in computational linguistics, or what is known as natural language processing (NLP). Abstractly, Markov models are nothing but a statistical representation of a particular system. The mathematical statistical representation of a given system is the heart of Markov theory. Markov models characterized by solid mathematical representation, which significantly promotes using it. No doubt, Markov models are mainly used in prediction and classification, to serve computational linguistics as well as other scientific applications. They include two main types: observable Markov models (for short, known as Markov models), and hidden Markov models (HMMs). Bearing in mind that the HMMs are an extension of the Markov models, to model certain applications. Aim: In this paper, I present a brief description of HMMs, as well as some HMMs-based NLP applications. Then, I shed light the researchers’ trend towards artificial neural networks (ANNs). Methods: the global digital libraries used to collect the topic related papers. The searching keywords focus on HMMs and ANNs. The collected papers filtered out to prepare the short list computational linguistics related papers. Then, the selected papers sorted based on published date to track the transformation process towards ANNs. Results: This study shows that the ANNs have been significantly and promising used in NLP tasks. Simultaneously, the recent NLP-related research shows that HMMs have less contribution for such tasks. That is, the ANNs (especially deep learning) is the de-fact trend in NLP field. Recommendations: the NLP research community and the new linguistic researchers should highly consider this transformation.
AbuZeina, D. E. (2024). Language Processing: The Precedence of Neural Networks on the Account of Hidden Markov Models. An-Najah University Journal for Research - B (Humanities), 39(3), 155–164. https://doi.org/10.35552/0247.39.3.2325
[1]D. E. AbuZeina, “Language Processing: The Precedence of Neural Networks on the Account of Hidden Markov Models,” An-Najah University Journal for Research - B (Humanities), vol. 39, no. 3, pp. 155–164, Oct. 2024, doi: 10.35552/0247.39.3.2325.
AbuZeina, Dia Eddin. “Language Processing: The Precedence of Neural Networks on the Account of Hidden Markov Models.” An-Najah University Journal for Research - B (Humanities), vol. 39, no. 3, Oct. 2024, pp. 155–64. Crossref, https://doi.org/10.35552/0247.39.3.2325.
1.AbuZeina DE. Language Processing: The Precedence of Neural Networks on the Account of Hidden Markov Models. An-Najah University Journal for Research - B (Humanities) [Internet]. 2024 Oct;39(3):155–64. Available from: http://dx.doi.org/10.35552/0247.39.3.2325
AbuZeina, Dia Eddin. “Language Processing: The Precedence of Neural Networks on the Account of Hidden Markov Models.” An-Najah University Journal for Research - B (Humanities) 39, no. 3 (October 2024): 155–64. https://doi.org/10.35552/0247.39.3.2325.
حوسبة اللغة: ما بين هيمنة الشبكات العصبية وتراجع نماذج ماركوف المخفية
المؤلفون:
معلومات المقال
2024-01-25
2024-07-19
2024-10-11
155 - 164
الكلمات الإفتتاحية
- Machine learning
- Artificial Neural Networks
- Features
- Hidden Markov Models
- Natural Language Processing
- Classifiers
- Computational Linguistics
الملخص
خلفية الدراسة: منذ اكتشافها في بدايات القرن الماضي، حظيت نماذج (ماركوف) على قبول واستحسان الباحثين، واستخدمت في مجالات متعددة، وكان لها حظ وافر ونصيب بارز من الأبحاث المتعلقة بحوسبة اللغة، أو ما يعرف (بـ معالجة اللغات الطبيعية). يُعد التمثيل الإحصائي الرياضي للعمليات العشوائية الأساس الذي بنيت عليه نظرية ماركوف إذ تعتمد على أساس رياضي متين، منحها شهرة واسعة، وجعلها محط أنظار الباحثين لفترة طويلة، الأمر الذي أحدث نقلة نوعية في عمليات التنبؤ والتصنيف، وفتح المجال لحوسبة اللغة وغيرها من العلوم، مثل الرعاية الصحية، والتسويق، وأسواق المال والاعمال، والتنبؤات الجوية الخ. وتشمل نماذج ماركوف نوعين رئيسين: نماذج ماركوف الظاهرة (اختصاراً يطلق عليها نماذج ماركوف)، ونماذج ماركوف المخفية. ورغم أن النوع الأول أفاد في مجالات بحثية كثيرة، إلا أنه لم يلبّ جميع الاحتياجات، فكانت نماذج ماركوف المخفية امتدادا لها، وتلبية لحاجة شريحة أوسع وأنماط مختلفة من المسائل البحثية. إلا أن السنوات الأخيرة شهدت توجهاً كبيراً نحو استخدام الشبكات العصبية الاصطناعية سواء في مجال حوسبة اللغات وفي غيرها من المجالات. أهداف الدراسة: تهدف هذه الدراسة الى رصد عملية انتقال الباحثين التحول نحو استخدام الشبكات العصبية الاصطناعية، وأثر ذلك على نماذج ماركوف المخفية. منهجية الدراسة: تم الاستعانة بقواعد البيانات الرقمية ومصادر الأبحاث الالكترونية لجمع أكبر عددٍ ممكن من الأوراق العلمية التي تتعلق بنماذج ماركوف المخفية والشبكات العصبية الاصطناعية، ثم فرزت هذه الأوراق لاختيار قائمة قصيرة من الأوراق النوعية المتعلقة بحوسبة اللغات، ثم صنفت الأوراق المختارة حسب فترة النشر، للخروج بتصور يوضح عملية الانتقال نحو الشبكات العصبية الاصطناعية. النتائج: تشير نتائج هذه الدراسة إلى أن العقد الأخير شهد تراجعاً في استخدام نماذج ماركوف المخفية، مقابل زيادة الاهتمام بالشبكات العصبية الاصطناعية (خاصة التعلم العميق)، ويمكن القول إن الشبكات العصبية الاصطناعية تهيمن اليوم على الأعمال البحثية الجديدة في مجال حوسبة اللغات وغيرها من المجالات. الخلاصة والتوصيات: إن التوجه الجديد نحو استخدام الشبكات العصبية الاصطناعية يفرض على الباحثين الجدد مواكبة هذا الانتقال، والاطلاع على نتائجه، والمساهمة فيه.
AbuZeina, D. E. (2024). Language Processing: The Precedence of Neural Networks on the Account of Hidden Markov Models. An-Najah University Journal for Research - B (Humanities), 39(3), 155–164. https://doi.org/10.35552/0247.39.3.2325
[1]D. E. AbuZeina, “Language Processing: The Precedence of Neural Networks on the Account of Hidden Markov Models,” An-Najah University Journal for Research - B (Humanities), vol. 39, no. 3, pp. 155–164, Oct. 2024, doi: 10.35552/0247.39.3.2325.
AbuZeina, Dia Eddin. “Language Processing: The Precedence of Neural Networks on the Account of Hidden Markov Models.” An-Najah University Journal for Research - B (Humanities), vol. 39, no. 3, Oct. 2024, pp. 155–64. Crossref, https://doi.org/10.35552/0247.39.3.2325.
1.AbuZeina DE. Language Processing: The Precedence of Neural Networks on the Account of Hidden Markov Models. An-Najah University Journal for Research - B (Humanities) [Internet]. 2024 Oct;39(3):155–64. Available from: http://dx.doi.org/10.35552/0247.39.3.2325
AbuZeina, Dia Eddin. “Language Processing: The Precedence of Neural Networks on the Account of Hidden Markov Models.” An-Najah University Journal for Research - B (Humanities) 39, no. 3 (October 2024): 155–64. https://doi.org/10.35552/0247.39.3.2325.
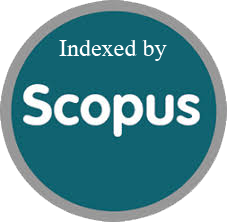
Since 2019
Cite Score (Scopus): 0.5
Time to First Decision: 7 Days
Submission to Acceptance: 60 Days
Acceptance to Publication: 10 Days
Acceptance Rate: 20%
Call for Papers:
Special Issue on
Innovative Assessment in the Age of AI: Strategies for Quality
Why should you
Publish With Us?
An-Najah National University
Nablus, Palestine
Nablus, Palestine
- P.O. Box
- 7, 707
- Fax
- (970)(9)2345982
- Tel.
- (970)(9)2345560
- (970)(9)2345113/5/6/7-Ext. 2628
- [email protected]
- EIC
- Prof. Waleed Sweileh